Simple Info About How To Deal With Multicollinearity
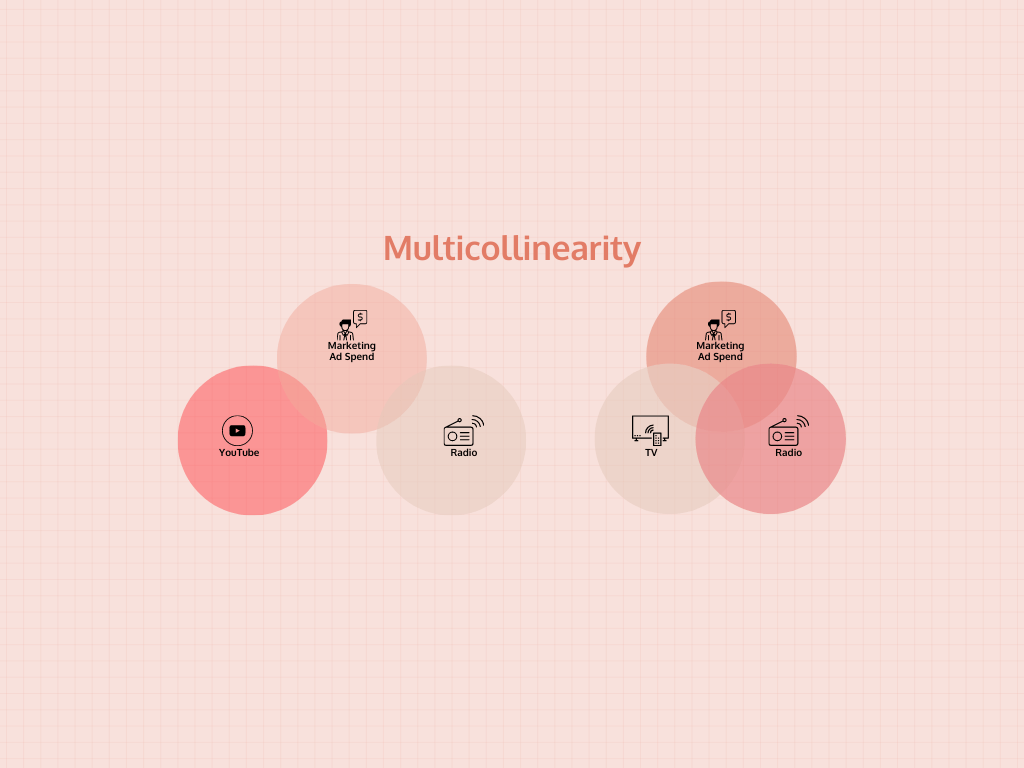
We can find out the value of.
How to deal with multicollinearity. Removing one of the variables from the model. 1 there are many ways to handle multicollinearity without having to sacrifice interpretability (as you correctly stated in your post). If x1 = total loan amount, x2 = principal amount, x3 = interest amount.
The good news is that it is not always mandatory to fix the multicollinearity. How to deal with multicollinearity? We have various statistical techniques that measure the.
Introduction multicollinearity, a common issue in regression analysis, occurs when predictor variables are highly correlated. Multiple linear regression let’s take an example of loan data. To reduce the amount of multicollinearity found in a statistical model, one can remove the specific variables.
Ridge regression can also be used when data is highly. It makes it hard to interpret of model and also. How to measure multicollinearity there are two popular ways to measure multicollinearity:
7 techniques to handle multicollinearity that every data scientist should know 1.) correlation matrix:. (1) compute a coefficient of multiple determination for each. Using principal components regression (pcr).
Correlation coefficient the pearson’s correlation coefficient metric directly evaluates the strength of the relationship between two variables. Multicollinearity (or collinearity) occurs when one independent variable in a regression model is linearly correlated with another independent variable. How to detect multicollinearity.
Improper dummy coding when you change a categorical variable into dummy variables, you will have one fewer dummy variable than you had categories. Multicollinearity happens when independent variables in the regression model are highly correlated to each other. I showed how there are a variety of situations where you don’t need to deal with it.
The multicollinearity might not be. Introduction multicollinearity is often described as the statistical phenomenon wherein there exists a perfect or exact relationship between predictor variables.
How to deal with multicollinearity. The most common way to detect multicollinearity is by using the variance inflation factor (vif), which measures the. We can deal with multicollinearity by:
This article navigates through the. It all depends on the primary goal of the regression model. One way to address multicollinearity is to center the predictors, that is substract the mean of one series from each value.